Data Governance and Data Quality have similarities, but they also differ. In this article we talk about these similarities and differences in detail.
Data offers great value to companies because of the competitiveness and differentiation they allow. However, they are under constant observation and analysis from a legal point of view, because of the regulations to which they are subject, which demand security, integrity and control. It is obvious that any company dealing with data must have a data governance policy that is the basis for a data management that can solve problems with the flow of data and reliability.
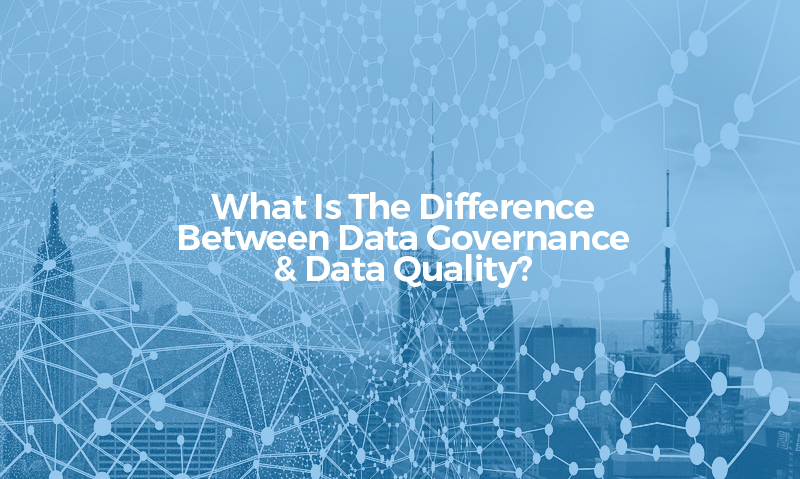
Data governance could be defined as "the exercise of shared authority, control and decision-making over the management of data assets". Data management, in turn, is the implementation of data policies.
It is important not to confuse any of these concepts with Master Data Management, which consists of achieving the integration of an organization's master data so that they can be used across different systems and departments.
Why is it important?
Many times, data governance policies appear as the company grows and has more data that allows it to be more competitive, identify points for improvement, develop products, improve the customer experience, etc., although the main reasons to develop a data governance policy are regulations and risk, so the company must have high quality data. In fact, many regulations check compliance by looking at the data security aspect.
Thus, the goal of data governance is to ensure the integrity of data assets through processes and procedures, standardization of systems, and creation of consistent data distribution policies.
Principles of data governance
- Integrity. That all the processes to which the data are submitted have as one of the objectives to maintain the integrity of the data.
- Life cycle. We must be able to follow the trace of the data, from its origin, through all its states, processes and destination.
- Security. We must watch over the privacy and confidentiality of the data by defining levels and profiles and the blocking of authorisations.
- Quality. Processes must be defined, controlled and improved in order to keep the completeness, accuracy, consistency, conformity, reliability and uniqueness of the data intact.
- Data lineage. We must be able to trace data to increase the reliability of business intelligence and minimize risks.
- Support the mission of data governance. Data governance is something that must have the full support of the company. We must ensure data accessibility, availability, quality, consistency, verifiability and security. Only then can we ensure absolute control over data assets.
- Establish rules applicable to data outside databases. We must establish data owners who are responsible for their management and plans in the short, medium and long term.
- Data storage. To maximize query agility, we must specify the storage of data, both in terms of location and volume.
Current status of the situation
On October 16, an event was held in Madrid focused on data management called Data Management Summit. The event included a round table discussion on data governance. The participants related their experiences around data management projects and their vision of the degree of maturity of the Spanish economoy in relation to this topic. From the questions raised at the round table, it was concluded, among other things, that although company management is already thinking about data governance, there is still not much budget dedicated to this aspect and the necessary organisational culture to successfully execute projects has not been implemented in general.
What is data quality?
Data are of quality when they meet a series of objectives: accuracy, relevance, completeness and understanding, but, above all, when they satisfy the requirements of the use to which they are to be put. Thus, companies have good quality data when they can use it to determine the needs of customers and serve them efficiently, although it is true that this vision is already becoming obsolete. Now companies are starting to focus on ensuring data quality across the board. From here, we conclude that data quality is the state of quality - or not - of the information elements available to a company.
In order to obtain quality data, several processes can be carried out, such as:
- Cleaning
- Standardization
- Profiling
- Geocoding
- Monitoring
Relationship with data governance
Data quality and data governance converge in processes aimed at ensuring the credibility and integrity of data. Furthermore, in order to comply with legislation and regulations —which is part of data governance— data must be of high quality.
Data quality focuses on the data itself, on its use and status, while data governance focuses on the authority that governs them and on maintaining control over it. In other words, the questions we have to ask ourselves in order to determine each of these questions are different, because their point of view and focus on the data are different. For example, a possible question to determine the state of data governance policy in the company could be "who is responsible for what aspects of the data?" and another for data quality would be "how useful are my data in the company?".
These two concepts differ in the framework in which they are situated, although both are essential for the subsequent management of master data needed to achieve a transversal integration in the organization.