Discover the top 10 trends in data and artificial intelligence for 2025. Explore how innovation in AI and data will transform the technology industry.
The world of data analytics and artificial intelligence (AI) is an ever-evolving and fast-moving world.
As we look ahead to 2025, major technology consultancies predict several trends that will significantly transform and shape the data and AI landscape.
Among these, we see a prominence of Large Language Models (LLM) and the increasing importance of data quality management strategies.
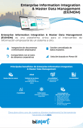
Data Quality Framework Datasheet
Check the technical capabilities of Data Quality Framework in the solution's datasheet.
Want to discover the trends that will shape the world of data and AI in 2025? Keep reading!
Here are the 10 trends set to dominate next year and how data and AI teams can adapt:
1. Transforming Data Infrastructure with LLMs
Large Language Models (LLMs) have revolutionized technology in recent years. In 2025, this trend will continue, driving increased demand for data and necessitating new data architectures, such as vector databases.
The integration of data analytics and automation will become standard across all levels of data infrastructure, enhancing data management and optimizing value for end users. Adopting LLMs will not only bring technological changes but also require rethinking current data management strategies. Companies will need to invest in infrastructure and training to fully leverage these advanced tools.
Transitioning to vector databases and other AI infrastructure components will be crucial to staying competitive in an increasingly AI-driven marketplace.
2. Data Teams Becoming Software Teams
The most advanced data teams are beginning to manage their assets as true data products, a trend known as Data as a Product (DaaP). This involves implementing product requirements, documentation, sprints, and service level agreements (SLAs) for end users.
As the value of these data products increases, data teams will be managed more like critical product teams. This evolution requires a cultural shift within organizations.
Data teams must adopt agile methodologies and development practices used in software engineering, including project management tools, version control systems, and code review processes.
The goal is to improve the quality and efficiency of data product development, ensuring they meet business standards and expectations.
3. Convergence of Software Engineering and Data
Software engineering and data are merging.
By 2025, no major software development will be launched without considering artificial intelligence and enterprise data. Engineers will need a deep understanding of data to create AI models that deliver continuous and meaningful value.
This convergence implies greater collaboration between software engineers and data scientists. Multidisciplinary teams will be essential to address complex challenges at the intersection of software and data. Software engineers will need to acquire data science skills, while data scientists must become familiar with software development best practices.
4. Rise of Retrieval Augmented Generation (RAG)
Retrieval augmented generation (RAG) will become essential for improving enterprise AI products.
As teams face contextual data challenges, the need for clean and curated data will become more apparent.
Organizations will develop best practices around RAG to create more robust and reliable AI products.
Implementing RAG will enable companies to improve the accuracy and relevance of their AI models. Using contextual and personalized data, models will generate more accurate and useful results for end users. This will enhance the user experience and increase confidence in AI solutions, encouraging wider adoption across different industries.
5. Deployment of Enterprise-Ready AI Products
By 2025, operationalizing AI products will be a priority.
Data teams will adopt more sophisticated practices to develop AI products focused on solving real business problems and creating value, rather than integrating technology for its own sake.
Creating enterprise-ready AI products requires a systematic and rigorous approach. Teams must conduct extensive testing to ensure the reliability and robustness of models before implementation. Additionally, establishing ongoing monitoring and maintenance processes is crucial to ensure AI products remain effective and relevant over time.
6. Data Observability for AI and Vector Databases
Ensuring data quality is essential for the success of generative AI.
Data teams will need robust data quality management and data observability strategies to detect, resolve, and prevent data issues in dynamic environments.
These solutions must prioritize efficient problem resolution and the resilience of streaming infrastructures supporting AI.
Data observability involves continuously monitoring data quality and proactively identifying potential problems. This includes implementing advanced data analysis and visualization tools and techniques. By enhancing visibility and control over data, organizations can ensure their AI models operate with accurate and reliable data, minimizing the risk of errors and biases.
Explore the features of Data Quality Framework, a solution that automates the validation of enterprise data quality through a comprehensive data observability system.
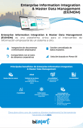
Data Quality Framework Datasheet
Check the technical capabilities of Data Quality Framework in the solution's datasheet.
7. Miniaturization of Big Data
Advancements in hardware are blurring the lines between commercial and enterprise solutions.
By 2025, data teams will use in-memory databases to manage and transfer data sets more efficiently. These quickly deployable solutions will enable rapid scaling and enterprise-level functionality.
The miniaturization of big data will also allow greater flexibility in data management. Companies can tailor their data infrastructures to specific needs, optimizing resource use and reducing costs.
Additionally, processing large volumes of data in memory will facilitate the development of real-time analytics applications, improving responsiveness and data-driven decision-making.
8. Prioritizing Right-Sizing
Balancing the use of more data with reducing cloud infrastructure costs will be a key focus in the coming years. Tools for monitoring metadata and right-sizing data utilization will be invaluable.
In 2025, organizations will seek to optimize their infrastructures to maximize AI's impact without incurring excessive costs.
Right-sizing involves identifying and eliminating inefficiencies in data and compute resource usage. Enterprises will need to invest in monitoring and data analysis tools to continually assess and adjust their infrastructure.
This proactive approach will not only reduce costs but also improve the performance and scalability of AI solutions.
9. Popularization of Apache Iceberg
Apache Iceberg, a data lake table format developed by Netflix, is becoming a standard solution for many organizations.
It provides structured and cost-effective storage that can be queried by different data processing engines. The adoption of Iceberg and its alternatives is expected to grow in 2025.
Apache Iceberg's popularity stems from its ability to manage large volumes of data efficiently.
Its support for multiple processing engines allows for greater flexibility and scalability in data management. Additionally, its optimized design facilitates real-time data access and analysis, which is crucial for artificial intelligence and advanced analytics applications.
10. Partial Return to the Office
As more companies implement back-to-office policies, the telework debate continues.
While some teams will continue to work from home, others will return to the office. Companies will need to balance the need for flexibility with the benefits of in-person collaboration.
Returning to the office can enhance collaboration and team synergy. However, it is important for organizations to implement flexible policies allowing employees to combine remote and in-person work.
This approach will improve employee satisfaction and productivity, and help companies attract and retain talent in a competitive labor market.
Conclusion
Data and AI trends for 2025 indicate an evolution toward greater integration, sophistication, and efficiency.
Transforming data infrastructure, converging software and data engineering, and implementing enterprise AI products will be critical to fully leverage the opportunities presented by the future of data and artificial intelligence.
Adaptability and innovation will be key to success in an ever-changing environment.
Organizations investing in the training and development of their teams, as well as implementing advanced infrastructure and technologies, will be best positioned to lead in data and AI in 2025 and beyond.