Hospital exitus percentages have been a healthcare indicator for a long time. Now with new technology this indicator can get a new, deeper perspective.
The percentage of hospital exitus (hospital mortality) is an indicator used to measure the quality of health care. Although mortality is affected by many different factors, such as illness, accessibility, etc., hospital mortality is useful to measure the effectiveness of health care and is used to take measures regarding the management of the centre.
Thus, the analysis of clinical data will allow us to know the information referring to the quality of hospital care, as well as to identify patterns and offer us a solid basis for taking management decisions.
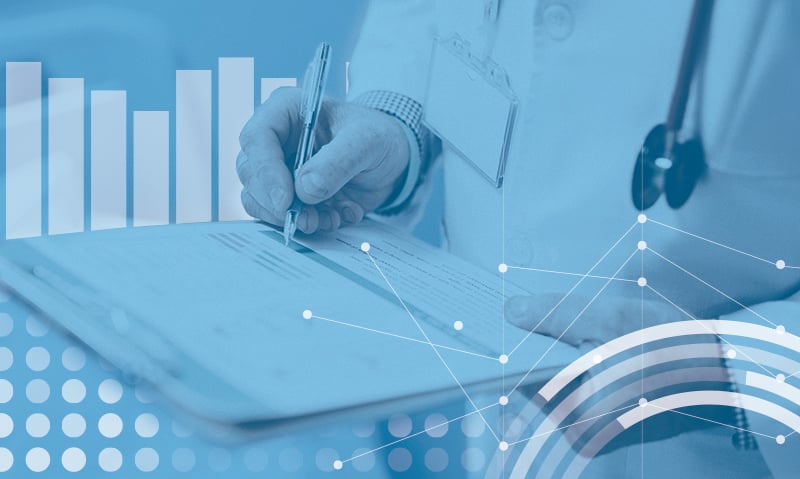
In addition, the use of data in clinical management can offer many advantages:
Discover unexpected patterns of exitus
As we have previously mentioned, knowing the percentage of hospital exitus, as well as the causes or reasons that surround it, is very important to evaluate the quality of care, as well as to make decisions. Analyses of hospital mortality are nothing new. However, with the introduction of technology these analyses take a new, deeper perspective, so that it is possible to detect patterns of exitus that were not visible before. This allows decision-making regarding clinical practice, as well as the administration of drugs or treatments and their relationship to underlying pathologies or comorbidity.
Accelerating diagnosis
Diseases present themselves in different ways in each patient. Comorbidity, age, sex, etc. can affect symptoms, so it is difficult for a doctor to offer a definitive diagnosis safely and quickly, as it is not humanly possible to know all the forms in which diseases are presented. However, computers can have all this information, which makes them a great support tool for doctors. In this way, it is possible to speed up the diagnosis process, which translates into early detection and treatment of diseases. In turn, this means better quality of life, greater chance of cure and recovery and a reduction in care costs.
Diagnostic support
Continuing with the use of data in the field of diagnosis, machine learning models allow new approaches to diagnosis by suggesting possibilities that are beyond the knowledge of a particular physician, especially in the most complicated and rare cases, in which errors often occur.
Likewise, with machine learning, new ways of detecting pathologies can be designed, such as X-ray readings, ECG, cytologies, teledermatology, etc.
Expected evolution
Technology not only gives us a broader view of the past and present, but also allows us to make predictions based on solid information from the data. Thus, the incorporation of technology facilitates the task of predicting the evolution of a disease or treatment for physicians. This allows doctors to stop relying on statistics and generalizations and to make assumptions based on reliable, contrasted and secure information thanks to the data.
Treatment evaluation
The use of technology can facilitate the choice and follow-up of treatments. Whether it is because doctors face very rare diseases or because it is not possible for them to keep absolutely up to date with all existing treatments, technology can be a tool to support that choice taking into account the specific circumstances of each patient and each disease, side effects, allergies, etc.
Technology is also useful in less practical fields within healthcare. Machine learning is very useful for clinical research and the monitoring of the effects of new drugs or new processes. Thanks to technology, it can be easy for researchers to detect patients who are eligible to participate in research.
In the field of detection of drug effects and consequences, there is a unique case. García Cantero, a prestigious ICT analyst, tells it in the technological portal TICbeat. In this instance, the big data analysis made it possible to detect that a drug, an analgesic, that was being administered with apparently positive results was causing an increase in deaths due to misuse and the harmful effects that had not been detected during the creation of the product and that the studies that were being carried out could not identify. Specifically, taking this analgesic was found to greatly increase the likelihood of having a heart attack. Thus, the use of the data on this occasion could explain why many deaths happened and prevent many more.
Limitations of machine learning for clinical practice
New technologies have been fully introduced in all fields and medicine and clinical practice are no exception. This applies to all the forms these professions take, from the most bureaucratic aspects, with clinical coding, to the most practical, such as diagnosis or even surgery. This gives medical and hospital staff the opportunity to focus more on the patient, as the more mundane tasks can be relegated to the machines. However, this is very difficult without quality data that allows us to work quickly and safely.