How do we transform data into business value? Discover what we do with data and the steps and processes of our data processing flow.
Nowadays no one questions the value of data, which has become an essential asset for companies. However, the value of data is neither incidental nor contingent. Data in its original format is like an unpolished diamond. Unlocking its value involves processing it, treating it and transforming it. In short, a long process that involves multiple tasks, technologies and disciplines.
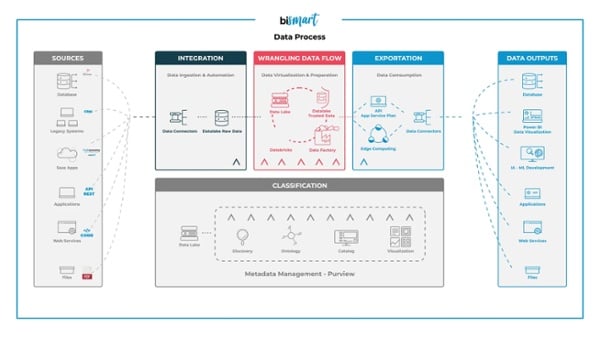
Think about diamonds. Diamonds come from stones. To turn a stone into a diamond, the stone must be cut and polished by experts. With data happens exactly the same.
The value of data in its original format and habitat is minimal and certainly insufficient for a company to take advantage of it to make better data-driven decisions.
We often say that data is the basis for business decision making. However, this statement is not entirely true. It is not the data itself that helps companies improve their business, but the knowledge it contains and that is hidden behind layers and layers of waste that needs to be removed. Data, like diamonds, must go through a process of polishing and cutting to be useful.
At Bismart we have been transforming raw data into business value for years. Some people are unaware or unaware of the process and the journey that data must go through to be transformed into value.
What do we do with data? Data processing
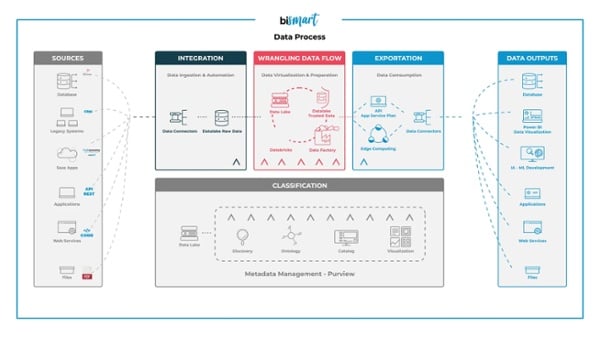
1. Data extraction
Today, data resides in multiple spaces known as data sources. Over the years, data sources have multiplied in the same way that data has.
Locating all data sources can be a daunting task, as now data is everywhere: analytic platforms, emails, databases, files of all kinds, social networks, etc.
At Bismart we have the ability to extract and collect data from any type of source, prioritizing the origin sources that may contain useful data:
- Structured and unstructured databases
- Legacy systems
- SaaS applications
- Other apps
- Internal or external web services
- Files in any format
2. Data ingestion
Once the data have been located and extracted from their original sources, they must be unified and integrated into a single space. Beyond their storage in a single database, what is really important about this process is that the data must be processed and transformed so that it can later be analyzed as a whole. Therefore, data is stored in a data lake, a type of database that stores raw data.
The process of bringing data together is known as data integration and is linked to system integration.
2. Data classification
Once the data is integrated, it must be classified. A first classification of macrodata —large volumes of data— is carried out to remove useless or unnecessary data. This is known as data cleansing.
This stage of the process also includes data mapping: metadata is aggregated and merged so that it can be mapped. That is, classified, identified and sorted. In our raw macro dataset we will find a lot of duplicate data that is repeated in multiple files with different nomenclature, format, etc. This data must be identified and aggregated into a master table so that systems can understand that it is the same information.
On the other hand, data is organized according to business needs: by subject area, by purpose, by time period, and so on. For this, data management and data governance are really important. Data management is essential and encompasses every stage of the data flow involved in data processing. Data governance and data management tasks are typically performed with Azure Pureview, a software that facilitates data administration and control and enables data scientists to build a holistic, real-time map of the overall data landscape through automated processes.
Basically, data classification consists of cross-referencing, sorting and mapping the data to give it meaning and make it intelligible.
3. Data transformation and processing: Wrangling Data Flow
The term "data wrangling" is used to describe the process of cleaning and transforming data. When raw data has been collected and stored in the data lake, it is time to prepare it —clean it, transform it and sort it— so that it can later be used in business intelligence projects.
Useful data, already classified, sorted and mapped, is extracted from the data lake and organized in data engineering and data science tools such as Azure Data Factory, which allows data transformation, sorting, aggregation, performing pipelines and dataflows, etc.
Data preparation also includes another tool: Azure Databricks. Databricks allows us to explore, execute and transform Big Data (structured and unstructured). Its main functionalities are the transformation, preparation and analysis of data that will later be transferred to other platforms for consumption. It is a key environment for analytics and the transformation of data into actionable information.
Both Data Factory and Databricks are intermediate services between the sources of origin of the data and its final destination.
4. Data exportation and consumption
Once the data is ready to be activated, it is exported to its final or temporary destination. Depending on the intended use of the data, it will be exported to a platform or another.
Data can be stored in consumption databases —usually a data warehouse— where it will be archived for its future use.
It can also be consumed by BI and data analytics tools such as Power BI, where the data will be analyzed and transformed into stories through data visualization and the creation of corporate reports and dashboards.
We can also export data to other types of artificial intelligence, machine learning and deep learning platforms, or be consumed by APIs, web services or transformed into files.
Data exportation also involves processes such as edge computing, a distributed computing model that brings computing and data storage as close as possible to the destination source to speed up processing and response times.
The extraction, transformation and loading of data can be summarized as an ETL or ELT process. However, the data flow required to extract value from available assets is much more comprehensive than an ETL process and requires complementary operations.
From the stone to the diamond: How to turn data into business value
Bismart's specialty is the transformation of data into business insights and actionable value that allows companies to optimize their processes, make better business decisions, increase their productivity or design better customer strategies, among many other things.
For all this to happen, data must go through a process that includes multiple sciences, involves different professional profiles and requires different technologies.
In this article we have described the most significant steps of a typical data processing flow. However, data processing can vary according to the needs of each company. In fact, it should. Not all companies have the same needs and, obviously, the data flow must be adapted to the characteristics and objectives of each corporate scenario.
At Bismart we are specialists in finding the best option and the most cost-effective process for you to leverage data and transform it into business intelligence and business value. We adapt to any type of environment and design the most efficient and profitable process for you.